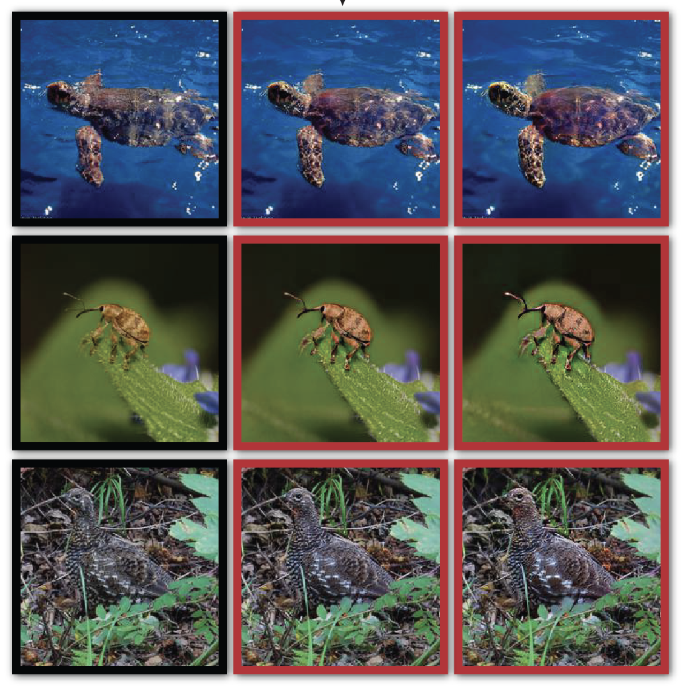
jan 30, 2025
Morgan Talbot presenting at Vision Journal Club
Morgan will be presenting the paper “L-WISE: Boosting Human Image Category Learning Through Model-Based Image Selection and Enhancement.”
For a concise summary, please see the project website. The paper explores ways to enhance visual category learning in humans by applying adversarially trained ANNs as models of visual perception.