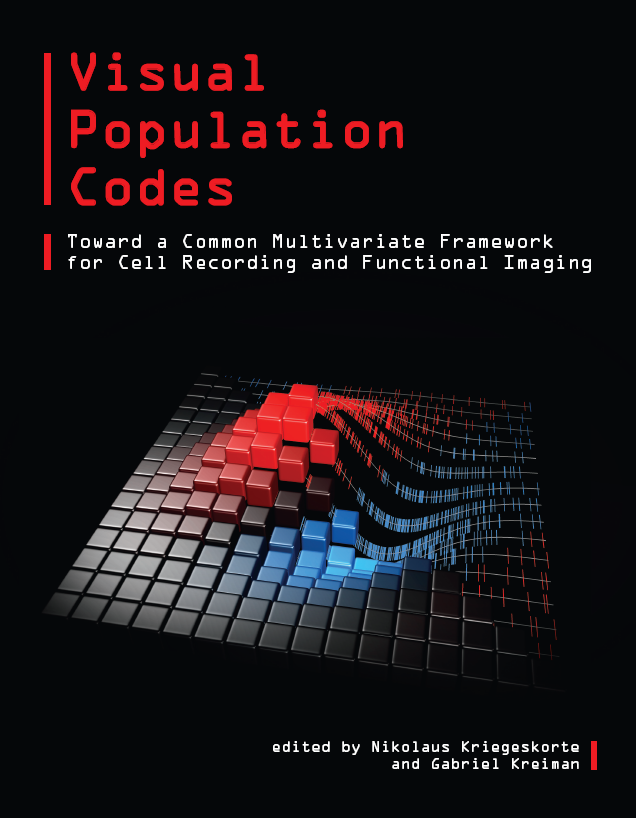
2011
A multivariate approach to understand visual representations by neuronal populations
Edited by Nikolaus Kriegeskorte and Gabriel Kreiman
Contributed chapters by Simon Thorpe, Sheila Neirenberg, Jasper Poort, Arezoo Pooresmaeili, Pieter Roelfsema, Yukiyasu Kamitani, Kendrick Kay, Jack Gallant, Shinji Nishimoto, Thomas Naselaris, Michael Wu, Anitha Pasupathy, Scott Brincat, Conor Houghton, Jonathan Victor, Hans Op de Beeck, Chou Hung, James DiCarlo, Marieke Mur, Nikolaus Kriegeskorte, Andrew Connolly, Ida Gobbinni, James Haxby, Dwight Kravitz, Annie Chan, Chris Baker, Dirk Walter, Diane Beck, Fei-Fei Li, John Dylan Haynes, Karl Friston, Kendra Burbank, Gabriel Kreiman, Jed Singer, Ethan Meyers, Stefano Panzeri, Robin Ince, Philipp Berens, Nikos Lothetis, Andreas Tolias.
Table of Contents
Introduction [Nikolaus Kriegeskorte and Gabriel Kreiman]
Vision is a massively parallel computational process, in which the retinal image is transformed over a sequence of stages so as to emphasize behaviorally relevant information (such as object category and identity) and deemphasize other information (such as viewpoint and lighting). The processes behind vision operate by concurrent computation and message passing among neurons within a visual area and between different areas. The theoretical concept of “population code” encapsulates the idea that visual content is represented at each stage by the pattern of activity across the local population of neurons. Understanding visual population codes ultimately requires multichannel measurement and multivariate analysis of activity patterns. Over the past decade, the multivariate approach has gained significant momentum in vision research. Functional imaging and cell recording measure brain activity in fundamentally different ways, but they now use similar theoretical concepts and mathematical tools in their modeling and analyses.
With a focus on the ventral processing stream thought to underlie object recognition, this book presents recent advances in our understanding of visual population codes, novel multivariate pattern-information analysis techniques, and the beginnings of a unified perspective for cell recording and functional imaging. It serves as an introduction, overview, and reference for scientists and students across disciplines who are interested in human and primate vision and, more generally, in understanding how the brain represents and processes information.
1. Grandmother cells and distributed representations [Simon Thorpe]
It is generally accepted that a typical visual stimulus will be represented by the activity of many millions of neurons distributed across many regions of the visual cortex. However, there is still a long-running debate about the extent to which information about individual objects and events can be read out from the responses of individual neurons. Is it conceivable that neurons could respond selectively and in an invariant way to specific stimuli—the idea of “grandmother cells”? Recent single-unit recording studies in the human medial lobe seem to suggest that such neurons do indeed exist, but there is a problem, because the hit rate for finding such cells seems too high. In this chapter, I will look at some of the implications of this work and raise the possibility that the cortical structures that provide the input to these hippocampal neurons could well contain both highly distributed and highly localist coding. I will discuss how a combination of STDP and temporal coding can allow highly selective responses to develop to frequently encountered stimuli. Finally, I will argue that “grandmother cell” coding has some specific advantages not shared by conventional distributed codes. Specifically, I will suggest that when a neuron becomes very selective, its spontaneous firing rate may drop to virtually zero, thus allowing visual memories to be maintained for decades without the need for reactivation.
2. Strategies for finding neural codes [Sheila Nirenberg]
A critical problem in systems neuroscience is determining what the neural code is. Many codes have been proposed—coarse codes, fine codes, temporal correlation codes, and synchronous firing codes, among others. The number of candidates has grown as more and more studies have shown that different aspects of spike trains can carry information (reviewed in Averbeck and Lee, 2004; Oram et al., 2002; Victor, 1999; Borst and Theunissen, 1999; Johnson and Ray, 2004; Theunissen and Miller, 1995; Nirenberg and Latham, 2003; Shadlen and Newsome, 1994; MacLeod, Backer, and Laurent, 1998; Bialek et al., 1991; Nirenberg et al., 2001; Parker and Newsome, 1998; Romo and Salinas, 2001; Dhingra et al., 2003; Gawne, Richmond, and Optican, 1991). Here we present a strategy to reduce the space of possibilities. We describe a framework for determining which codes are viable and which are not, that is, which can and cannot account for behavior. Our approach is to obtain an upper bound on the performance of each code and compare it to the performance of the animal. The upper bound is obtained by measuring code performance using the same number and distribution of cells that the animal uses, the same amount of data the animal uses, and a decoding strategy that is as good as or better than the one that the animal uses. If the upper-bound performance falls short of the animal’s performance, the code can be eliminated. We demonstrate the application of this approach to a model system, the mouse retina.
3. Multineuron representations of visual attention [James Poort, Arezoo Pooresmaeili, Pieter Roelfsema]
Recently techniques have become available that allow simultaneous recording from multiple neurons in awake behaving higher primates. These recordings can be analyzed with multivariate statistical methods, such as Fisher linear discriminant analysis or support vector machines to determine how information is represented in the activity of a population of neurons. We have applied these techniques to recordings from groups of neurons in visual primary cortex (area V1). We find that neurons in area V1 do not only code basic stimulus features, but also whether image elements are attended or not. These attentional signals are weaker than the feature-selective responses, and it might be suspected that the reliability of attentional signals in area V1 is limited by the noisiness of neuronal responses as well as by the tuning of the neurons to low-level features. Our surprising finding is that the locus of attention can be decoded on a single trial from the activity of a small population of neurons in area V1. One critical factor that determines how well information from multiple neurons is combined is the correlation of the response variability, or noise correlation, across neurons. It has been suggested that correlations between the activities of neurons that are part of a population limit the information gain, but we find that the impact of these noise correlations depends on the relative position of the neurons’ receptive fields: the correlations reduce the benefit of pooling neuronal responses evoked by the same object, but actually enhance the advantage of pooling responses evoked by different objects. These opposing effects cancel each other at the population level, so that the net effect of the noise correlations is negligible and attention can be decoded reliably. We next investigated if it is possible to decode attention if we introduce large variations in luminance contrast, because luminance contrast has a strong effect on the activity of V1 neurons and therefore may disrupt the decoding of attention. However, we find that some neurons in area V1 are modulated strongly by attention and others only by luminance contrast so that attention and contrast are represented by largely separable codes. These results demonstrate the advantages of multi-neuron representations of visual attention.
4. Decoding early visual representations from fMRI ensemble responses [Yukiyasu Kamitani]
Despite the wide-spread use of human neuroimaging, its potential to read out perceptual contents has not been fully explored. Mounting evidence from animal neurophysiology has revealed the roles of the early visual cortex in representing visual features such as orientation and motion direction. However, non-invasive neuroimaging methods have been thought to lack the resolution to probe into these putative feature representations in the human brain. In this chapter, we present methods for fMRI decoding of early visual representations, which find the mapping from fMRI ensemble responses to visual features using machine learning algorithms. First, we show how early visual features represented in ‘sub-voxel’ neural structures could be predicted, or decoded, from ensemble fMRI responses. Second, we discuss how multi-voxel patterns could represent more information than the sum of individual voxels, and how an effective set of voxels can be selected from all available voxels that leads to robust decoding. Third, we demonstrate a modular decoding approach in which a novel stimulus, not used for the training of the decoding algorithm, can be predicted by combining the outputs of multiple modular decoders. Finally, we discuss a method for neural mind-reading, which attempts to predict a person’s subjective state using a decoder trained with unambiguous stimulus presentation.
5. Understanding visual representation by developing receptive-field models [Kendrick Kay]
To study representation in the visual system, researchers typically adopt one of two approaches. The first approach is tuning curve measurement, in which the researcher selects a stimulus dimension and then measures responses to specialized stimuli that vary along that dimension. Stimulus dimensions can range from low-level dimensions, such as contrast, to high-level dimensions, such as object category. The second approach is multivariate pattern classification, in which the researcher collects the same type of data as in the tuning-curve approach but uses these data to train a statistical classifier that attempts to predict the dimension of interest from measured responses. This approach has recently become quite popular in functional magnetic resonance imaging (fMRI). In this chapter, we argue that the tuning curve and classification approaches suffer from two critical problems: first, these approaches presuppose that individual stimulus dimensions can be cleanly isolated from one another, but careful consideration of stimulus statistics reveals that isolation is in fact quite difficult to achieve; second, these approaches provide no means for generalizing results to other types of stimulus. We then describe receptive-field estimation, an alternative approach that addresses these problems. In receptive-field estimation, the researcher measures responses to a large number of stimuli drawn from a general stimulus class and then develops receptive-field models that describe how arbitrary stimuli are mapped onto responses. Although receptive-field estimation is traditionally associated with electrophysiology, we review recent work of ours demonstrating the application of this technique to fMRI of primary visual cortex. The success of our approach suggests that receptive-field estimation may be a promising direction for future fMRI studies.
7. Population coding of object contour shape in V4 and posterior inferior temporal cortex [Anita Pasupathy, Scott Brincat]
When we see an object, its shape is encoded by neural activity patterns in the ventral pathway of visual cortex. It is well established that successive stages in the ventral pathway encode increasingly complex and invariant object structure. However, it has been difficult to elucidate exactly how object structure is encoded. Critical questions include: What attributes or dimensions of object shape are encoded at each stage? How are these neural codes distributed across populations of neurons? How is the neural code at one stage transformed into the neural code at the next stage? Recent single-neuron recording experiments in two successive ventral pathway stages—area V4 and posterior inferotemporal cortex (PIT)— shed light on these questions. Results show that V4 and PIT neurons encode the shapes and spatial relationships of multiple contour fragments along object boundaries. Complete information about an object is distributed across populations of neurons that span the contour fragment domain, such that object shape can be decoded with linear basis function analyses. More complex PIT tuning for multi-fragment configurations may be synthesized by feedforward summation of V4 inputs combined with recurrent network processes. These findings provide a preliminary understanding of shape coding in neural populations, and they point to future experiments examining network-level transformations between successive population codes.
8. Measuring representational distances: the spike-train metrics approach [Conor Houghton, Jonathan Victor]
Since 1926 when Adrian and Zotterman reported evidence that the firing rates of somatosensory receptor cells depend on stimulus strength, it has become apparent that a significant amount of the information propagating through the sensory pathways is encoded in neuronal firing rates. However, while it is easy to define the average firing rate for a cell over the lengthy presentation of a time-invariant stimulus, it is more difficult to quantify how temporal features of spike trains relate to the stimulus. With a real, experimental, data set, for example, extracting a time-dependent rate function is model dependent since calculating it requires a choice of a binning or smoothing procedure. The spike train metric approach is a framework that distills and addresses these problems. One family of metrics are “edit distances” that quantify the changes required to match one spike train to another; another family of metrics first maps spike trains into vector spaces of functions. Both these metrics appear successful in that the distances calculated between spike trains seems to reflect the differences in the information the spike trains contain. Studying the properties of these metrics illuminates the temporal coding properties of spike trains. The approach can be extended to multi-neuronal activity patterns, with the anticipation that it will prove similarly useful in understanding aspects of population coding.
9. The role of categories, features, and learning for the representation of visual object similarity in the human brain [Hans Op de Beeck]
Inferior temporal (IT) cortex contains visual object representations at the interface between perception and cognition. Although IT has been intensively studied in monkeys and humans, IT representations of the same objects have never been compared between the species and IT’s role in categorization is not well understood. Here we presented monkeys and humans with the same images of real-world objects and measured the IT response pattern elicited by each image. Two images similar in the monkey-IT representation tend also to be similar in the human-IT representation. Moreover, IT response patterns form category clusters, which match between the species. The clusters correspond to animate and inanimate objects; within the animate objects, faces and bodies form subclusters. Within each category, IT distinguishes individual exemplars and the within-category exemplar similarities also match between monkey and human. Our findings suggest that primate IT across species may host a common code, which combines a categorical and a continuous representation of objects.
10. Ultrafast decoding from cells in the macaque monkey [Chou Hung, James DiCarlo]
In a glance, a fraction of a second, our minds capture the visual scene. We distinguish life from the inanimate, faces from background, the familiar and the unfamiliar. The seeming ease of visual recognition belies the difficult of reading out the underlying neural code, much less the reconstruction of its mechanism in silico. The computational difficulty of visual recognition is the combination of selectivity for specific objects and invariance across changes in viewing conditions. Both properties have been shown to a limited extent for single neurons in the macaque anterior inferior temporal (AIT) cortex at the end of the ventral visual pathway. An ongoing challenge is determining whether and how the activity of a population of such neurons is sufficient to encode object category and identity. We recently showed, based on independent recordings from several hundred recording sites, that the brief (~12 msec) activity of a small population of AIT neurons is indeed sufficient to support recognition across changes in object size and position. Remarkably, the combination of selectivity and tolerance also exists for novel objects. This chapter will review the motivations and outcomes of that study and discuss recent work and major issues in developing effective read-out from IT cortex.
11. Representational similarity analysis fo object population codes in humans, monkeys, and models [Nikolaus Kriegeskorte, Marieke Mur]
12. Three virtues of similarity-based multivoxel pattern analysis: an example from the human object vision pathway [Andrew Connolly, Ida Gobbini, James Haxby]
We present an fMRI investigation of object representation in the human ventral vision pathway highlighting three aspects of similarity analysis that make it especially useful for illuminating the representational content underlying neural activation patterns. First, similarity structures allow for an abstract depiction of representational content in a given brain region. This is demonstrated using hierarchical clustering and multidimensional scaling (MDS) of the dissimilarity matrices defined by our stimulus categories—female and male human faces, dog faces, monkey faces, chairs, shoes, and houses. For example, in ventral temporal (VT) cortex the similarity space was neatly divided into face and non-face regions. Within the face region of the MDS space, male and female human faces were closest to each other, and dog faces were closer to human faces than monkey faces. Within the non-face region of the abstract space, the smaller objects—shoes and chairs—were closer to each other than they were to houses. Second, similarity structures are independent of the data source. Dissimilarities among stimulus categories can be derived from behavioral measures, from stimulus models, or from neural activity patterns in different brain regions and different subjects. The similarity structures from these diverse sources all have the same dimensionality. This source independence allowed for the direct comparison of similarity structures across subjects (n = 16) and across three brain regions representing early, middle, and late stages of the object vision pathway. Finally, similarity structures can change shape in well-ordered ways as the source of the dissimilarities changes—helping to illuminate how representational content is transformed along a neural pathway. By comparing similarity spaces from three regions along the ventral visual pathway, we demonstrate how the similarity structure transforms from an organization based on low-level visual features—as reflected by patterns in early visual cortex—to a more categorical representation in late object vision cortex with intermediate organization at the middle stage.
13. Investigating high-level visual representations: objects, bodies, and scenes [Dwight Kravitz, Annie Chan, Chris Baker]
Human functional magnetic resonance imaging (fMRI) studies have revealed cortical regions selectively responsive to particular categories of visual stimuli (e.g., faces, body parts, objects, and scenes). However, it has been difficult to probe beyond this category selectivity to investigate more fine-grained representations, in part because traditional fMRI designs make implicit assumptions about the structure of those representations. Here, we take advantage of the flexibility of ungrouped event-related designs and the power of representational similarity analysis to directly investigate within-category representations of object, body parts, and scenes. This approach enables us to elucidate how the structure of these representations relates to categorization, individuation, and the complex relationship between the two. Responses from up to ninety-six conditions were analyzed using an iterative split-half correlation method allowing us to simultaneously investigate categorical structure (by grouping stimuli based on their response-pattern similarities) and individuation (by comparing the similarity between individual stimuli). First, we show that object-selective cortex contains distinct representations of the same objects in different positions. Second, we find that body-selective cortex contains distinct representations of different types of body parts. Further, those representations are strongest for body parts in their commonly experienced configuration. Finally, we show that scene-selective cortex contains strong representations of individual scenes and further categorizes scenes based on their expanse (open, closed—the boundary of the scene). In each case, the flexibility afforded by condition-rich ungrouped-events design and representational similarity analysis allowed us to design data-driven experiments capable of revealing surprising and counterintuitive aspects of high-level representations.
14. To err is human: correlating fMRI decoding and behavioral errors to probe the neural representation of natural scene categories [Dirk Walther, Diane Beck, Fei-Fei Li]
Vision science has made tremendous progress in understanding how the brain processes various components of our visual world. Much of this progress is owed to the advent of functional magnetic resonance imaging (fMRI), a non-invasive neuroimaging method that allows for the imaging of activity in the whole brain. Indeed, fMRI has enabled the mapping of several important visual areas in the human brain, for instance, retinotopic visual cortex including primary visual cortex and extrastriate regions (Engel, Rumelhart et al. 1994), the lateral occipital cortex for object perception (Malach, Reppas et al. 1995), the fusiform face area (Kanwisher, McDermott et al. 1997), and the parahippocampal place area (Epstein and Kanwisher 1998). In these seminal studies univariate statistics (each voxel was treated independently) was employed to produce maps of functional activity. It has now been shown that considering the specific pattern of activity of multiple voxels in response to visually presented objects allows for finer distinctions between categories of objects, leveraging the distributed nature of the representation of objects in the human brain (Haxby, Gobbini et al. 2001). This discovery has spurred a surge of studies applying multi-voxel pattern analysis (MVPA) techniques to many questions in visual neuroscience and beyond.
15. Decoding visual consciousness from human brain signals [John-Dylan Hanes]
Despite many years of research on the neural correlates of consciousness (NCC), it is still unclear how conscious experience arises from brain activity. Many studies have treated consciousness as an all-or-nothing phenomenon—for example, by comparing conscious and unconscious processing of the same features. However, the important question how the specific contents of consciousness are encoded in the human brain has often been ignored. It is frequently assumed that the contents of consciousness are encoded in dedicated neural carriers or “core NCCs,” one for each different aspect of conscious experience. However, identifying such core NCCs is a difficult task because many regions correlate with every aspect of conscious experience. For this reason it is important to formulate empirical criteria that allow assessing whether a brain region that is involved in processing of a certain feature (say, color or motion) is also directly involved in encoding this feature in conscious experience. Now, the approach of multivariate decoding provides a novel framework for studying the relationship between consciousness and content-selective processing in more detail. It allows to directly investigate the mapping between brain states and the contents of consciousness. Most important, decoding can be used to test the important criterion of “injectivity”: A brain region can be said to encode a particular type of experiences only if it is possible to decode these experiences in a loss-free fashion from activity patterns in that region. This approach makes it possible to assess how conscious experience is encoded in the brain and how the encoding of sensory information is affected when it enters awareness.
16. Probabilistic codes and hierarchical inference in the brain [Karl Friston]
This chapter addresses the nature of population codes by assuming that the brain has evolved to enable inference about the causes of its sensory input. This provides a principled specification of what neuronal codes have to represent: they have to encode probability distributions on the causes of our sensations. We attempt to address how these distributions are encoded by casting perception as an optimization problem. We propose a model in which recognition arises from the dynamics of message passing among neuronal populations. The model is consistent with our knowledge of intrinsic and extrinsic directed connections in the brain. The model equates perception with the optimization or inversion of internal models of how sensory input is generated. Given a generative model that relates environmental causes to sensory signals, we can use generic approaches to model inversion. This corresponds to mapping from the sensory signals back to their causes—that is, to recognize stimuli in terms of neuronal activity patterns that represent the causes of sensory input. The model’s hierarchical and dynamical structure enables it to recognize and predict sequences of sensory events. We first consider population codes and how they are constrained by neuronal recognition schemes. We then show that the brain has the necessary infrastructure to implement recognition under a particular form of probabilistic code (a Laplace code). We present a simulation of a bird brain that generates and recognizes birdsongs. We conclude with a simple neuroimaging experiment that tests some of the theoretical predictions entailed by this approach in the context of the human visual system.
17. Introduction to the anatomy and function of visual cortex [Kendra Burbank, Gabriel Kreiman]
18. Introduction to statistical learning and pattern classification [Jed Singer, Gabriel Kreiman]
Here we provide a brief introduction to the field of statistical learning with multiple references to the mathematical literature. We discuss the setting of the learning problem, how supervised learning problems can be formulated and addressed and several algorithms to learn from data including Fisher linear discriminant, nearest neighbors, support vector machines. The discussion is linked to a neurophysiological recordings from ensembles of neurons.
19. Tutorial on pattern classification in cell recordings [Ethan Meyers, Gabriel Kreiman]
We outline a procedure to ‘decode information’ from multivariate neural data. We assume that neural recordings have been made from a number of trials in which different conditions were present, and our procedure produces and estimate of how accurately we can predict the presents of these conditions in a new set of data. We call this estimate of future prediction accurately the ‘decoding/readout accuracy,’ and based on this measure we can make inferences about what information is present in the population of neurons and also on how this information is coded. The steps we cover to obtain a measure of decoding accuracy include: 1) formatting the neural data, 2) selecting a classifier to use, 3) applying cross-validation to random splits of the data, 4) evaluating decoding performance through different measures, and 5) testing the integrity of the decoding procedure and significance of the results. We also discuss additional topics including: 1) how to examine questions about neural coding using feature selection, different binning schemes, and different classifiers and 2) how to evaluate whether invariant/abstract variables are contained in a dataset by training and testing a classifier using data recorded under different conditions.
20. Tutorial on pattern classification in functional imaging [Marieke Mur, Nikolaus Kriegeskorte]
21. Information-theoretic approaches to pattern analysis [Stefano Panzeri, Robin Ince]
In this chapter, we review an information-theoretic approach to the analysis of simultaneous recordings of neural activity from multiple locations. This approach is relevant to the understanding of how the central nervous system combines and evaluates the messages carried by different neurons. We review how to quantify the information carried by a neural population and how to quantify the contribution of individual members of the population, or the interaction between them, to the overall information encoded by the considered neuronal population. We illustrate the usefulness of the information-theoretic approach to neural population coding by presenting examples of its applications to simultaneous recordings of multiple spike trains and/or of local field potentials (LFPs) from visual and somatosensory cortices.
22. Local field potentials, BOLD, and spiking activity: relationships and physiological mechanisms [Philipp Berens, Nikos Logothetis, Andreas Tolias]
Code links
Chapter 18 [Singer, Kreiman]
Introduction to statistical learning and pattern classification
We provide a non-exhaustive list fo links that can help users interested in implementing and using some of the ideas in this chapter.
Numerical recipes (The art of scientific computing)
Center for biological and computational learning at MIT
Literature and links to SVM software
Kreiman lab code repository
EEGLAB: Matlab toolbox for ICA and other analyses on multichannel data
Ethan Meyer’s toolbox
Fabrizio Gabbiani’s spike train analysis techniques
LibSVM toolbox
Stefano Panzeri’s toolbox